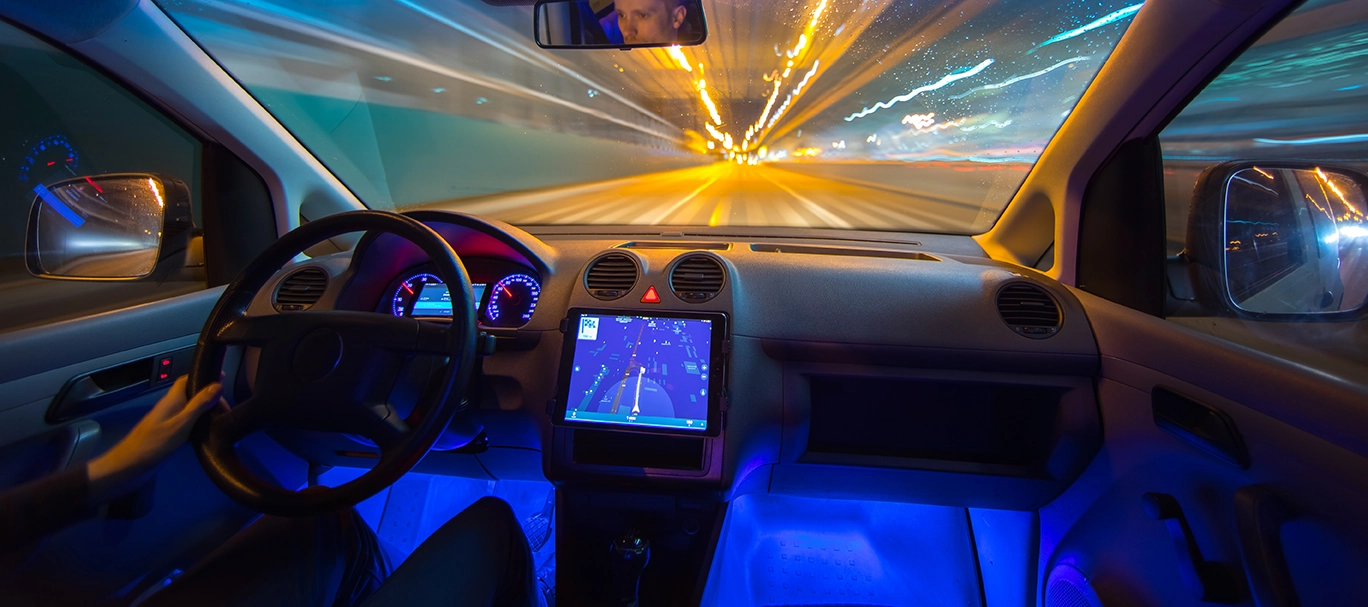
Welcome to the Centric3 series on machine learning, in this article, we explore Autonomous Systems—perceiving, learning, and making decisions in dynamic environments. Autonomous Systems represent a convergence of artificial intelligence, robotics, and sensor technologies, paving the way for machines that can navigate and interact with the world independently. Join us as we navigate the core concepts, applications, and challenges that define the landscape of Autonomous Systems.
Introduction
Introduction to Autonomous Systems
Defining Autonomy
Autonomous Systems refer to machines or agents that can perform tasks and make decisions without continuous human intervention. These systems have the ability to perceive their environment, learn from experiences, and adapt their behavior to achieve specific goals.
Components of Autonomous Systems
Sensors: Autonomous Systems rely on various sensors, such as cameras, LiDAR, radar, and other environmental sensors, to perceive and understand their surroundings.
Decision-Making Algorithms: Advanced algorithms, often powered by machine learning, enable Autonomous Systems to make decisions based on the information gathered from sensors and prior experiences.
Actuators: Actuators, such as motors and servos, allow Autonomous Systems to interact with and manipulate their environment based on their decision-making.
Autonomy Levels
Levels of Autonomy
Level 1: Driver Assistance: Basic assistance features, such as cruise control, fall into this category, where the human driver retains control.
Level 2: Partial Automation: The vehicle can control both steering and acceleration, but the human must remain engaged and monitor the environment.
Level 3: Conditional Automation: The system can manage most aspects of driving, but the human may need to intervene in certain situations.
Level 4: High Automation: The vehicle can operate autonomously in specific conditions or geofenced areas, with no human intervention required.
Level 5: Full Automation: The system can handle all driving tasks under all conditions, and no human driver is needed.
Notable Applications
Applications of Autonomous Systems
Self-Driving Vehicles
In the automotive industry, Autonomous Systems power self-driving vehicles that aim to enhance safety, efficiency, and convenience in transportation. Most notable in this space is obviously Tesla.
Drones
Autonomous Systems enable drones to navigate and perform tasks, including surveillance, package delivery, and agricultural monitoring. Most notable here is Amazon Drone Delivery.
Industrial Robots
In manufacturing and logistics, Autonomous Systems drive industrial robots that can perform tasks like assembly, welding, and material handling. Tesla also making strides here, video below.
Challenges
Challenges & Considerations
Safety & Liability
Ensuring the safety of Autonomous Systems raises questions of liability and accountability, especially in scenarios where decisions made by the system have significant consequences.
Ethical Decision Making
Autonomous Systems may face ethical dilemmas, such as deciding how to prioritize safety in emergency situations. Developing ethical frameworks for decision-making is an ongoing challenge. See the Centric3 article on Explainable AI for more information.
Future
Future Directions & Advancements
Multi-Agent Systems
Advancements in Autonomous Systems involve exploring scenarios with multiple agents operating collaboratively. Multi-Agent Systems enhance coordination and communication among autonomous entities.
Swarm Robotics
Swarm robotics takes inspiration from collective behavior observed in natural systems like ant colonies. Autonomous Systems working in swarms can achieve complex tasks through decentralized coordination.